Meta Unveils Llama 4 Scout and Maverick: Open-Weight Multimodal Models Built for Performance and Scale
Both models were distilled from Llama 4 Behemoth, a larger unreleased model with 288 billion active parameters.
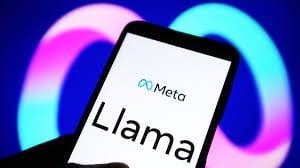
Meta has released two new open-weight multimodal AI models: Llama 4 Scout and Llama 4 Maverick. These models are available for download on llama.com and Hugging Face, and can be accessed through Meta AI on WhatsApp, Messenger, Instagram Direct, and the Meta AI website.
Llama 4 Scout is a 17 billion active parameter model built with 16 experts using a mixture-of-experts (MoE) architecture. It fits on a single H100 GPU and supports a 10 million token context window, enabling tasks like multi-document summarization and reasoning over large codebases. Meta says Scout outperforms models like Gemma 3 and Gemini 2.0 Flash-Lite, while remaining more efficient and scalable than previous Llama versions.
Llama 4 Maverick, also with 17 billion active parameters, includes 128 experts and 400 billion total parameters. It's intended for higher-end applications and rivals much larger models such as DeepSeek V3, with strong performance on reasoning and coding tasks. Meta says Maverick outperforms GPT-4o and Gemini Flash 2 on several benchmarks.
"Whether you’re a developer building on top of our models, an enterprise integrating them into your workflows, or simply curious about the potential uses and benefits of AI, Llama 4 Scout and Llama 4 Maverick are the best choices for adding next-generation intelligence to your products," Meta said in a blog post.
Both models were distilled from Llama 4 Behemoth, a larger unreleased model with 288 billion active parameters. Behemoth has already shown leading results on STEM tasks and influenced Scout and Maverick through a process called codistillation.
The models introduce architectural changes such as interleaved attention layers and training on diverse multimodal data. Meta used a streamlined fine-tuning process, reinforcement learning, and preference optimization to improve performance.
More announcements are expected at LlamaCon on April 29, including details on an upcoming Llama 4 reasoning model.